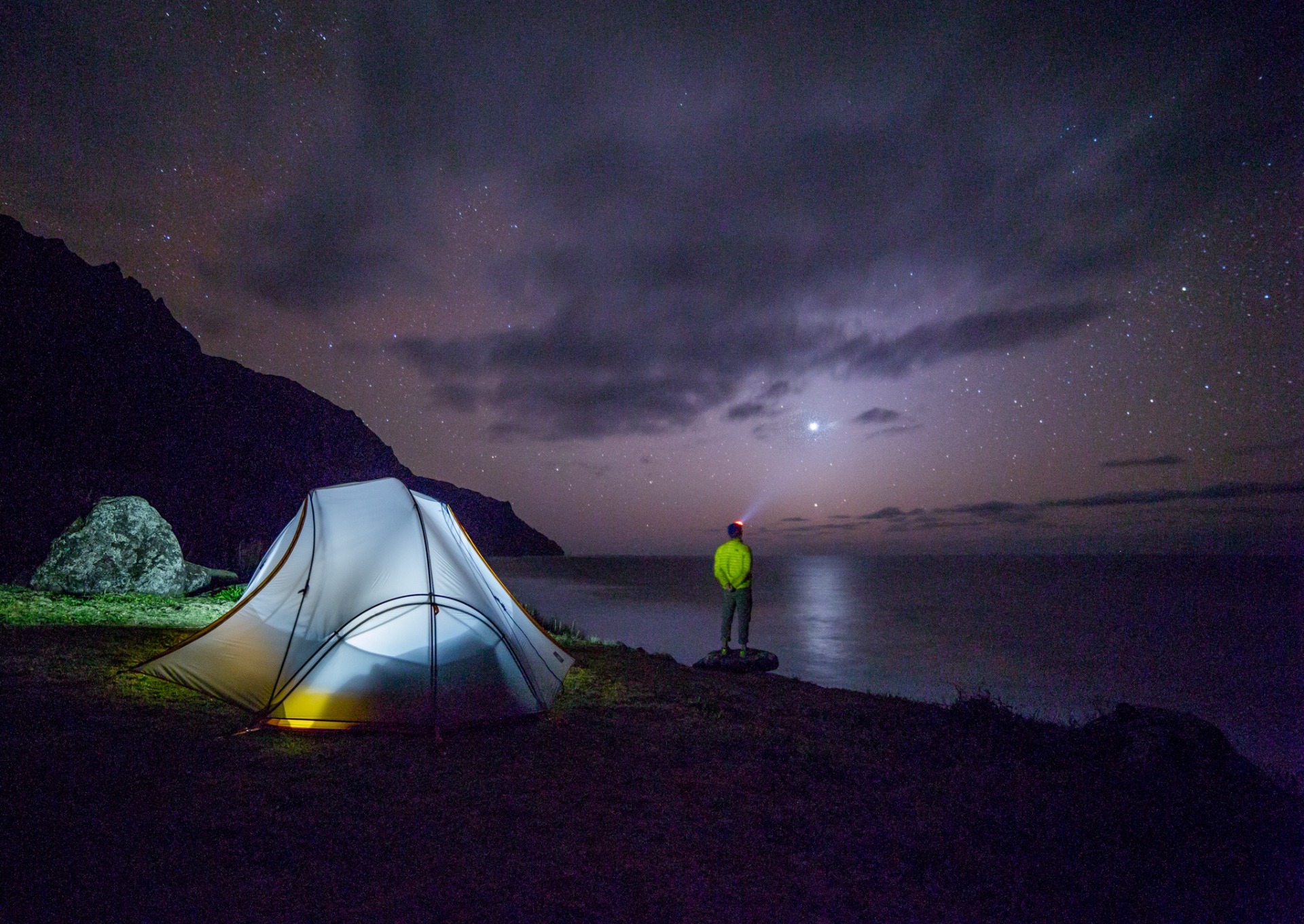
Internal Validity in Research: Definition, Threats, & Examples
Internal validity is one of the most crucial elements in experimental research, determining whether the results of an experiment can be confidently attributed to the manipulations made in the study, rather than to external factors. In other words, it ensures that the cause-and-effect relationship found between the independent and dependent variables is real and not the result of confounding or extraneous variables. This blog post will dive into the definition of internal validity, discuss the common threats to it, and provide examples to illustrate how researchers can maintain high internal validity in their experiments.
What Is Internal Validity?
Internal validity refers to the extent to which a study can establish a cause-and-effect relationship between the independent variable (the factor being manipulated) and the dependent variable (the factor being measured). High internal validity means that the results of the experiment are due to the treatment or intervention applied and not other confounding factors.
Why Internal Validity Matters
The importance of internal validity lies in its ability to ensure that the conclusions drawn from an experiment are accurate. Without high internal validity, an experiment cannot demonstrate a true causal relationship between two variables. For example, if you're testing whether coffee improves memory performance, a study with low internal validity may misinterpret other factors—such as time of day or participants' prior mood—as the cause of the observed effects. In simple terms, if your experiment has high internal validity, you can confidently say that the changes you observe are directly caused by your independent variable (the treatment) rather than some external or hidden factors.
How to Check for Internal Validity in Research
There are three key conditions that must be met to establish internal validity in a study:
- Covariation: The treatment and the response variable must change together. If the dependent variable shows changes after the manipulation of the independent variable, then there is covariation.
- Temporal Precedence: The cause (independent variable) must precede the effect (dependent variable). This ensures that the manipulation of the independent variable comes before any changes are observed in the dependent variable.
- Elimination of Confounding Variables: There should be no extraneous variables or confounding factors that could explain the observed effects. This is where researchers need to carefully control for outside influences that could potentially distort the findings.
Imagine you're testing whether drinking a cup of coffee improves memory. You randomly assign participants to two groups: one group drinks coffee (the treatment group) and the other drinks water (the control group). If the coffee group performs better on a memory test than the water group, you could hypothesize that coffee improves memory. However, if the experiment fails to account for the time of day or other variables, such as sleep patterns, you cannot confidently say that the coffee is the only factor affecting memory performance. This experiment, in that case, would have low internal validity.
Threats to Internal Validity
Several threats can undermine internal validity, particularly in experimental designs. These threats can come from both single-group and multi-group studies. Here's a look at some common threats and strategies to mitigate them. History refers to any external event that happens during the course of the study that can affect the outcomes. For example, if a participant's mood is influenced by an unexpected event like a political announcement, their responses may be affected, which could distort the results. To counteract history effects, researchers can conduct studies over shorter periods or create controlled environments where such external events are less likely to affect participants. Maturation involves natural changes that occur in participants over time. For instance, if a study measures cognitive performance over several months, the natural aging process or other developmental changes could affect results. A control group can help differentiate between changes due to the intervention and those due to natural progression. When participants are repeatedly tested, their performance may improve simply due to familiarity with the test rather than any real effect of the independent variable. Using different versions of the test or limiting the number of tests administered can reduce the impact of testing effects. Changes in the measurement tools or procedures used during the study can introduce bias. For example, if different researchers conduct interviews at different times with varying levels of experience, the results may not be comparable. Researchers can standardize all procedures and ensure that instruments are calibrated correctly throughout the study. Attrition occurs when participants drop out of the study, which can lead to biased results if those who drop out differ significantly from those who remain in the study. Tracking why participants drop out and using statistical techniques like intention-to-treat analysis can help manage this threat.
Countering Threats in Multi-Group Studies
In multi-group studies, such as when comparing different treatments or interventions, additional threats to internal validity may arise. Some of the most common threats include:
- Selection Bias: When participants are not randomly assigned to groups, there may be pre-existing differences between the groups that affect the results. Random assignment can help ensure that each group is comparable at the start of the study.
- Regression to the Mean: Extreme scores at the start of the study are likely to move closer to the average on subsequent tests, which may skew results. Random assignment helps ensure that extreme scores are equally distributed across groups.
- Social Interaction: Participants may communicate with each other between groups, which could influence the outcomes of the study. Blinding or isolating groups from one another can help reduce these effects.
- Attrition Bias: If participants drop out unevenly across groups, the results may become biased. Keeping track of participants and adjusting for attrition can help address this threat.
Examples of High Internal Validity
In a clinical trial testing a new medication, researchers randomly assign participants to either a treatment group or a placebo group. Both groups are followed over several months, with consistent measurement tools and protocols. The results show significant differences between the groups, suggesting that the medication has a real effect. This study has high internal validity because the researchers controlled for extraneous variables and used random assignment to ensure the groups were comparable. A study testing the effects of a cognitive training program on older adults randomly assigns participants to either a treatment group or a control group. The study uses a double-blind design, ensuring that neither the participants nor the researchers know which group each participant is in. The results show that the treatment group performs better on cognitive tasks, demonstrating the effectiveness of the cognitive training program with high internal validity.
Conclusion
Internal validity is vital for establishing a credible cause-and-effect relationship in experimental research. By understanding the various threats to internal validity and taking steps to mitigate them—such as using random assignment, standardizing procedures, and controlling for confounding variables—researchers can ensure that their study results are valid and reliable. High internal validity allows researchers to confidently draw conclusions about the effects of the independent variable on the dependent variable.
Frequently Asked Questions (FAQs)
1. What is internal validity? Internal validity refers to the degree to which a study accurately shows a cause-and-effect relationship between the independent and dependent variables, without interference from other variables.
2. How can I improve internal validity in my research? To improve internal validity, you can use random assignment, control extraneous variables, standardize measurement procedures, and use control groups.
3. What are common threats to internal validity? Common threats include history, maturation, testing effects, instrumentation, attrition, and selection bias.
4. What's the difference between internal and external validity? Internal validity focuses on whether the study accurately establishes cause-and-effect relationships within the study, while external validity refers to how generalizable the results are to other settings, people, or times.
5. Why is internal validity important in research? Internal validity ensures that the conclusions drawn from a study are credible and that the observed effects are due to the independent variable rather than confounding factors.